In this episode of The Discussion I’m joined by Josh Muncke - Commercial Director, Retail & Consumer at Faculty and Sam Gregory - Executive Director at Human Rights Organisation, WITNESS. We cover generative AI models, what they are and how they're changing the customer experience. Testing and iterating as a process, and how the field of prompt engineering is going to evolve. Also shallow fakes versus deep fakes, the novel ability to create new media content from existing data, the need for safeguards and transparency. And of course, what's next for GPT, and the next generation of models.
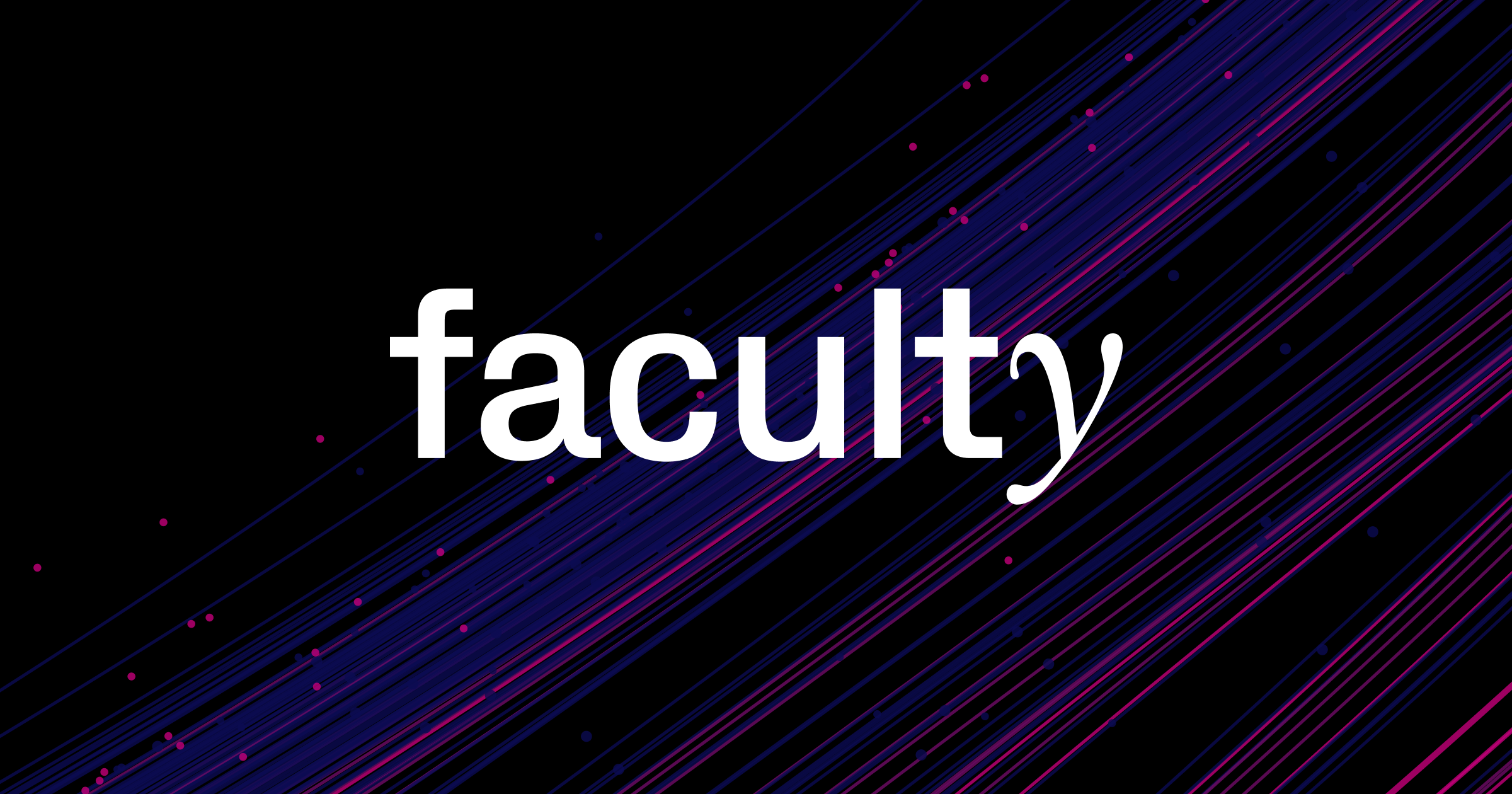
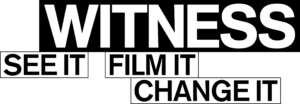
Transcript
Tracey Follows 00:20
Welcome to the Future of You. This week, I'm in conversation with two people who closely assess, monitor, and certainly in one case deploy AI in their everyday work. I talked with Josh Muncke, who is the Commercial Director of Retail and Consumer at Faculty, one of the UK is best known AI and decision intelligence companies. And Sam Gregory, Executive Director at Witness, the human rights organisation. I thought this would make a great combination. Or perhaps I should say, comparison, because what I discussed with Josh is the effect of AI on consumers and with Sam the effect of AI on citizens. Although of course, there is crossover now, because, as I would argue, in a digital world, the boundaries between consumer and citizen are fast disappearing. Look at it like this, once everything is a service, then everyone is a subscriber, no longer just a citizen or just a consumer. But maybe that's a thought for another day. For now, let me just say that this episode covers a lot of ground - generative models, what they are and how they're changing the customer experience, testing and iterating as a process, if you're working with them, and how the field of prompt engineering is going to evolve. Also shallow fakes versus deep fakes, the novel ability to create new media content from existing data, the need for safeguards and transparency. And of course, what's next for GPT, and the next generation of models. But first, is this where we all expected to be in 2023?
Josh, welcome to the show. Now, the last time we spoke was a while ago, back in 2020, when you very kindly helped me with a lot of research for my book, and we were talking about generative AI and its implications way back then. And we will return to prompt engineering in a moment and what you had to say about it, then. But I wondered if we could start by you telling me if this is where you expected us to be in 2023? So we've had GPT exploding onto the scenes, disrupting everything from the creative industries to education systems. We've had Italy banning its use, of course, as well as some legal firms, lately. We've had the pause letter, which we may come on to. And we've had the AI generated song by Drake in The Weekend that was not by Drake and The Weekend, actually, at all. So presumably, we're only at the beginning of all of this or are we? I'd love to get your take.
Josh Muncke 02:54
Great question. And I think, you know, even for those of us that have been kind of in the field for a while and thinking about AI and generative AI, I think many of us have been caught off guard by the pace that this technology has exploded into the public awareness. And it's hard to kind of sometimes remember that it was only less than six months ago, that the ChatGPT was kind of released into the public domain and how quickly that gained so many users. So I, you know, I thought we would have made some good progress in 2020. And GPT3 when it was originally released was that was a big step up in terms of capability and performance. But the pace that we've seen over the last six months, the amount of stuff that has happened, the amount of progress that's been made, but also the amount of awareness of this set of technologies and its capabilities, has moved far more quickly, in the last six months than I thought it might do. And it's it's interesting to think about how much of that is down to the technology itself. How much of that is down to interfaces for the technology and making these sorts of models more accessible for everyday people to interact with and get a feel for, and how much is due to, you know, regulators and legislators starting to wake up to the possible impact that these sorts of things are going to have on everyday life and the world around us.
Tracey Follows 04:27
Yeah, that's so interesting to hear people in the industry saying that even they are, in part surprised about the speed of this. And I guess it's the scale as well, isn't it? I mean, I mean, the scale of GPT and what open AI have done not to mention the cost, of course, but the trillions. I don't know it must be more than that, of words that have gone into this training data, I mean, just the sheer scale, can it continue to get bigger and bigger or have we seen almost the limits now that like large language model are we into sort of reinforcement learning or new ways of presenting this?
Josh Muncke 05:05
Yeah, I mean, that is a good question. Because I think up until now, we've certainly seen a kind of trend where bigger is better, and GPT4 or is certainly much bigger and certainly much better than GPT 3.5, or ChatGPT. But actually, it may be that we're starting to kind of hit the plateau of the improvements that you can get from just adding more data and more words into these models. And actually, that we see changes to the architecture of the models, or the reinforcement learning and human feedback steps on top of those models are actually the next wave of improvements. So that's how we're going to get the next the next level of improvements of these systems. And certainly, I know, Sam Altman has pretty much said as much fairly recently that we may kind of be topping out on just adding more data to GPT models. Others in the, other influences in the community have got even more fundamental challenges with GPT models altogether as a route to generalise intelligence. But yeah, I think the answer is probably probably a bit of both, like I think probably there is still value in, to be gleaned from from from bigger. But it might not be quite as as linear, as we've seen historically. And actually, we see maybe more of the improvements in the future coming from other approaches being added on top of those large models that really generate the next level of performance.
Tracey Follows 06:31
I was going to ask you actually, Faculty have got a partnership of some sort with Open AI, right, you should tell us about that. But also, what do you think about the market as a whole, obviously, we've had Google coming back with Bard and other competitors. And as you say, they might be slightly behind. Or maybe they're not? I don't know, it'd be really nice to have an overview from an insider about about the market overall.
Josh Muncke 06:53
I think market is in a really interesting space at the moment. As you said, Faculty are partnered with Open AI and our objective and the work that we do with our customers is all around helping them to use AI in a productive way and in a safe way. And so for us, when we were looking at the market, the right choice was to partner with the Open AI guys who we saw as having both the best capability but also the kind of most robust and safe capabilities when it comes to actually using these in a commercial or an enterprise context. But you know, the reality is, it is quite a volatile market. There are other players that are doing some really amazing stuff. The team at Anthropic, you mentioned the team at Google within the image space, you've got Stability and Midjourney, and recently Hugging Face released their open source, kind of chat, large language chat based language model. So it's moving really quickly. I think, overall, that's good. It's a good thing. We're seeing that having some, you know, spurring on innovation, and through competition, and also pricing. And we've seen some kind of dramatic shifts and drops in things like pricing, which, if you're a business looking to actually use this stuff, to do significant amounts of work in your business that makes a difference, and it opens up use cases that otherwise might be might be prohibitive. As to how it shakes out, you know, I can take a guess at the next six to 12 months. But beyond that, beyond that, it's it's kind of anyone's guess. It does seem like, you know, to some extent, there's there's two directions that that this could go - one which is kind of consolidation, where that the requirements for data size data volumes, training costs, essentially make it prohibitive for anyone but the very small number of vendors to have models that are truly like viable, usable, large language models or generalised models, in a sense that people could use. I think it's also interesting to think that not every use case, not every business needs the biggest large language model for everything. And so I think it's quite feasible that we see, you know, a bit of a tiered market where you have a small number of operators at the top of the pyramid offering these very large models, but then actually a much wider number of players offering small large language models. But for more maybe maybe more specialised, or use case specific applications.
Tracey Follows 09:15
Yes, that's fascinating. And you hinted at that when we spoke about this in in 2020, actually, that there would be like, services starting up, you know, to help companies with very bespoke ways to integrate this, which kind of brings us on nicely to the question I wanted to ask you, which was, you know, how are businesses operationalizing these transformers if indeed they are? Because that's one of the questions. Is anybody doing it well, is everybody experimenting? How are businesses and organisations doing that? I know you work with a lot, a lot of them. So perhaps you could give us a little bit of insight into that?
Josh Muncke 09:50
Sure. Yeah. So everyone's experimenting is the thing I'd say first, but I think there's very few executive teams that have not at least had the conversation about how might this be relevant to them either in a sense of a threat to how they run currently or as an opportunity of how they could operate. So every business I speak to is starting to think about this. Some are further forward and have started to deploy these things for particular use cases. And I think what we're seeing very much is this idea of, let's pick one or two places where we see a really strong fit between what the models can do, and what we need the use case to be able to do. And so the types of applications we're seeing there are places where one is a process that involves language, right, because these are language models. And so in general, they're going to be processes that involve large amounts of language. So things like a customer service or, or marketing, where language is a key part of the process, but also two really importantly, where there is an element of human in the loop or validation around that. And, yes, I think we are seeing kind of a push towards, you know, things like chatbots and fully automated services. But in general, we are seeing most businesses taking a slightly more thoughtful approach than just fully automating their customer experience using a large language model. And actually saying, we can use these to augment the way that our current teams operate, to make them more efficient, to make them be able to respond more quickly, or to be able to provide more context and make them better performing in the way that they respond or interact with customers. The power of these models to digest large amounts of data and then support human customer service agent, for example, is actually tremendous. And there's a business case all of its own, you don't need to fully automate your entire customer service team. And it probably is not the right time to make that level of relatively risky investment before you've validated the technology yourself. So yeah, I think lots of use cases that we're seeing are in that kind of you know customer engagement, customer understanding, customer service kind of space. And generally in a way in which is kind of augmentative rather than fully automated.
Tracey Follows 11:59
Now I know you you work a lot in the area of retail, retail and consumer, don't you? So can you tell us some of the types of consumer interactions or customer experiences that might be transformed by some of this tech?
Josh Muncke 12:12
Yeah, I think this is one of the really profound moment, actually, this kind of the birth of these sorts of generative models, is really profound in retail and consumer. And I think this is actually not a set of industries or sectors that is new to AI, and machine learning and data science. It is, you know, relatively common now for retailers to use AI machine learning methods to support with things like product search, or marketing personalization. But I think what's changed is the dynamic from kind of these more traditional narrow AI models to these generative models. So when we used to think of models, or machine learning is kind of moving up this pyramid of descriptive, to predictive, to prescriptive. So better understanding what happened in the past, to predicting what is going to happen in the future, to then telling me what the best action is to take, and maybe automating that. And ultimately, for kind of a retail or consumer sense, a lot of what that allowed you to do was was filter, filter and sort and rank things. So you've got a lot of products that you can show to a customer, what's the best one, I should show them? You have a number of offers or marketing messages that you could send to someone what's the best one to send to them? With generative AI, it kind of sits outside of that framework a little bit because no longer are you just filtering and ranking and sorting, you are generating new data. And so I think that really transforms how you might think about how you interact with a shop or a consumer company or your network operator, or really anyone where you have a kind of customer/provider a relationship with because rather than that organisation offering you a number of different experiences or products, and then the models filter that, actually, you might have an agent or a conversational AI that is actually able to generate something entirely new and bespoke and novel for you. Both in the way you search for it, interact with it, and potentially even in the product offering itself. And so I think we're seeing the start of that. But that's gonna take a little while for these sorts of businesses to reconfigure themselves. If indeed, some of them can. Moving from traditional retail view of the world to one which is totally bespoke, maybe even at the customer level,
Tracey Follows 14:22
God, I've got so many questions that I want to ask you about that. Where shall I start? Well, one of them is will this replace the whole idea of segmentation? Clients love, their very, very expensive segmentation models, and some of them are useful, and some of them aren't, I think, but that's what is kind of the was the industry standard, you know, a quite finessed segmentation model. What you're, you're explaining there is a completely different model and you don't go anywhere near segmentation, because you don't need to because you have personalization. So how will this evolve for companies to think?
Josh Muncke 14:57
Yeah, you know, segmentation is such an interesting one, because some versions of the world segmentation ends up being the tail that wags the dog and you see businesses get so religious almost about their segmentation that they kind of forget that it's surely only a means to an end of serving a customer in a slightly more nuanced or granular way. And so I think it's a really good question. We've seen, we've seen the evolution of kind of segmentation go from broad personas, male 25 to 35, based in London, down to more granular kind of behavioural based upon the types of activities you like to take through to kind of segment of one. But all of that is really just a means to an end, right, which is how do I better personalise an interaction, a homepage, a message, in a world in which that interface is now mediated by a model entirely, maybe you don't need to have that segmentation? And actually, the model carries a lot of the weight of that personalization work. So rather than you the retailer, having an incredibly granular segmentation that covers every single nuance and variety of customer behaviour, and then you use that to personalise your customer interactions for each individual person, actually, the model plays that role of understanding exactly what the customer needs, exactly what they're looking for, exactly how they like to purchase, and then pulls the right set of products, of offers, of messages from the bank, or generates them entirely to generate an experience that is truly personalised and totally bypasses that idea of having a very granular, rigid segmentation on which a decision is made. So that's a fundamental shift. That's just not how retailers think today. But you can imagine it being transformative when it comes to how a customer experience looks from now to 10 years in the future.
Tracey Follows 16:50
What about the people that don't want to interface if you like, with companies like this? So somebody who perhaps doesn't have a smartphone, doesn't want to speak to a chatbot, etc? Going back to like the segmentation, psychology, they don't have to all be old people! I think there's this thought that like, what people don't want to use this tech, I say all over the place. You know, some people do want to get really engaged in this and other people want the almost purely human interaction. What happens for the people that fall outside of the potential analysis by the model?
Josh Muncke 17:24
Yeah, I think basically, the good retailers will crack that nut and realise that personalization is not like hyper personalising every customer's experience, it's actually realising that some customers don't want that. And that, you know, actually, the right thing to do is to provide the balance, like use these things as tools that augment rather than a total replacement for how you did things in the past. That need and requirement for nuance and customer relationships is key. And I think we'll see differences play out across different sectors and different industries. You know, the luxury sector, you know, the relationship that customers have with a brand like Chanel, for example, is fundamentally different from, let's say, Tesco, right? They're both fantastic brands, but just the nature of the relationships and interactions that customers have with those two businesses are different. And then within those brands, the nature of the different customers, different customer relationships have will be fundamentally different. So I think the retailers and the brands that approach this thoughtfully will, will bake that into their plan and will avoid alienating kind of large, important portions of their customer base by forcing everyone into the same working kind of operating paradigm. But it just seems inevitable that there will be this kind of gradual change, and even for things like, you know, cashless and contactless payment, right? You know, we've seen a massive adoption and uptake of that over the last 10 or 15 years, but some people still want to use cash. There are retailers that have been possibly burned and found problems with the fact that they've kind of lost demand because they've not supported that others haven't made the kind of the trade off the business case, that actually, that's worth it. The costs of cash handling, it's, it's not worth it. And that's going to be case by case.
Tracey Follows 19:03
So again, that's back to having the human insight and understanding of the brand and how its customers want to engage, isn't it outside of the model really?
Josh Muncke 19:11
Yeah, I don't think we're yet at the point where we kind of say, you know, GPT, what's our business strategy?
Tracey Follows 19:18
I'm sure some people have plugged that in. [laughs]
Josh Muncke 19:20
I'm sure they have! But I don't know that I would want to run my business on that quite yet.
Tracey Follows 19:24
No exactly. I wanted to ask you about how this technology interfaces with other technology in a retail space? So for example, over the past few years, we've seen a lot of kind of, you know, try on, particularly in beauty, skincare, things like that, and obviously clothing, you know, try it on even, you know, with hairstyles and anything that's kind of cosmetic like that. How does that data feed in, if it does to a sort of an LLM or a personalization model that we've been discussing here? Do they integrate?
Josh Muncke 19:58
I think they very quickly will start to, and actually, you know, we've got so excited about large language models over the last four or five months, that we've kind of forgotten that really the space is more broadly about generative models. And that kind of imagery and video is, is a really big part of that, you know. It was, well, not long ago, we were talking about Midjourney and Dali and some of these amazing, amazing models. And, you know, the next wave, the next version of GPT will have kind of multimodal capability. And we'll see a lot tighter coupling between text and images, images and audio, audio and text. And, and so that's exciting. So, you know, what does that mean for how the customer experience looks like, for things like trying on or visualisation of clothing, or furniture or designs? I think really exciting, I think, really, really exciting. Over the last few weeks, we've been trying to decorate our dining room, which is the fastest path to an argument in my house is kind of interior decoration. And we were using GPT to kind of do some brainstorming around colour schemes and furniture types, and then took the outputs of that and put it into Dali to kind of generate some sample kind of examples of what that could look like. And that's kind of very rough and ready, quite rudimentary, but you don't have to think very hard at all to imagine how that process could look in a more industrialised way, when you shop online for furniture or for interior decoration. Or when you search indeed, for clothing. Where actually the process becomes far more personalised, far more individualised at a visual level and you're able to really design and create and cocreate what you want something to look like before you ever even try it on. And maybe even before it even exists. And it's kind of it's possible to imagine a world in which you're no longer picking from a catalogue of products. But actually, what's created is actually made for you, based upon that design process, that codesign process that is totally enabled by generative models, which take the form of image and and text.
Tracey Follows 22:01
Yeah, I think there's a sustainability argument that people apply to that where you're literally only producing a range of one. The thing that you chose to bring into reality from a from a virtual space, right, which is, again, just fascinating, really.
Josh Muncke 22:17
And also on the sustainability piece, I think its interesting as well, because there is the kind of what if we, what if we just produced what we have demand for, but also, what if we can eliminate, you know, the things like returns and orders that don't go ahead, because people have had the chance to really genuinely see how this item will look? We'll see how the, the thing will fit in a really, really visual, clear, precise, high fidelity way before they ever buy it. So I think clearly, there's a bit of a Metaverse kind of question to that as well. And like quite how that is mediated is a separate question. But I think really interesting how the shopping experience changes could have kind of profound sustainability impacts as well in terms of the things that we need to produce to satisfy potential demand.
Tracey Follows 23:06
So over the course of this podcast, in previous episodes, we've talked about the death of authenticity, and the new era of profilicity. And Hans-Georg Mueller was very good on this. And I've used his work in papers I've written to make the case that we are now as identities in a digital era in cyberspace, really the summation of our profiles. So actually, the information that is collected on us and the way we are seen by the tech platforms, has, by default, become our identity, in a sense. Are these generative AI models going to add to that, do you think? Do they feel even further profilicity and the death of authenticity, do you think>
Josh Muncke 23:50
I think, augment, you know, they augment and it's hard to, it's hard to come down on one side too strongly or another. I think, you know, this idea of identity is so interesting as it pertains to the consumer. And I'm certainly not the first person to say, you are what you buy, which is something which is increasingly true of people today, but it's pretty different from generations. Even, you know, our parents generations would maybe not have strongly, strongly define ourselves by the choices they make about the clothes they wear, the food they eat, the holidays, they go on. But increasingly, that is increasing. That is a really important part of how we view ourselves. I think what's really changing with generative models is that whereas in the past, you had systems that could filter down a set number of options and kind of recommend the one that might be the right fit for us. In a generative world, you might have systems that entirely cocreate an experience, an outfit, a meal just for us. And at that point, when you are who you buy. In some sense, your personality is kind of deeply interconnected, interconnected with that generation process. So you're no longer just using a dumb machine learning system to filter about the things that you don't want, and by the things that you do, the system is playing a really important role in helping you actually decide and create what you decide to purchase, whatever that is. And I think in that paradigm, in that world, that idea of identity then kind of gets wrapped up in this agent or this model. And that that presents a really interesting question as to who we are, as it pertains to what what we decide to consume.
Tracey Follows 25:25
So will they really know who we are? Or will they just know what we tend to buy and predict what we might buy next, and therefore, just some of the patterns that pertain to us rather than actually our name and who we are as a person?
Josh Muncke 25:40
Yeah, I think at some point, the difference between knowing who we are, actually, and knowing deeply enough our personal choices and preferences around everything we buy, the places that we go, may become a bit indistinguishable. Because at a certain point, you can represent someone well enough by the behavioural choices around how they decide to spend their money, that knowing their name, or their address, at that point, it kind of becomes a superfluous piece of detail. And that's really what I'm talking about. If that model is so deeply in tune with the way you like to purchase and consume, you know, media and clothing and, and the like, at what point does it become really an extension of you versus just an agent? That's an interesting question that I don't know the answer to.
Tracey Follows 26:28
I wanted to talk to you about prompt engineering and where you think we are with that. Obviously, you when I talked to you back in 2020, you talked to me about prompt engineering, where I didn't really know what it was then, of course, and you were saying that we might find GPT analysts and GPT specialists who exist purely to help organisations tune and optimise their in house versions of these models, across a variety of use cases. We may see organisations or individuals that specialise in producing content, such as marketing, specifically targeted for consumption of these algorithms. At the moment, people are all talking about prompt engineering. But there's another group of people saying, oh, that's just, that's just a stepping stone to something else. How important do you think it is that people understand, can do, can carry out prompt engineering to a high degree?
Josh Muncke 27:18
I don't know. Honestly, I really don't know. It sounds like I was kind of being quite prescient back in 2020. But actually, I'm sure I was just basically parroting some wisdom that I had read somewhere else at the time. But it's really interesting. And clearly, at the moment, there is, there are loads of people doing some extremely interesting stuff, when it comes to how you interact with these models in effective ways. And there's really interesting examples of that. There are people that are doing that, and the kind of red teaming side and finding out if you ask these models, in clever ways, they'll reveal things in ways that they were not supposed to, or potentially harmful information. That's a form of prompt engineering, there are people that are doing things like asking the models to think, step by step. And they find that if you do that, they find that actually, you tend to get better, more well reasoned, and more high quality responses out of these models. And then, of course, there's the kind of prompt engineering which is, you know, setting these models up to give them a clearer sense of what type of output you want, what type of response looks good, and what type of response looks bad, and how they should evaluate the inputs and how they should then generate outputs. So all of that kind of prompt engineering, I think is great. And actually, I've been amazed with how quickly again, my Twitter feed is filled up with people with amazing examples of kind of clever and creative prompts that have been produced. Again, I'm sure the OpenAI guys have talked a little bit about that as well and said, actually, that space of people, engineering prompts has moved way more quickly than they thought. And actually, I think probably the best prompt engineers, potentially don't even work for one of these companies, the large language model companies, they're kind of developers, they're external. How does the field evolve from here? Like I said, I don't know. One of the things I would say is, over time, there is clearly a need to kind of iterate on prompts. And for the businesses that are using large language models, they may find that actually, continuing to optimise the prompt over time, is one of the ways that they continue to make sure that the models can work very, very well. And, you know, there's lots of things that are happening in that space to allow the ongoing optimization of prompts, To a point where maybe the products themselves are not even human English. They're just kind of like vectors, like in some embedding space, that are being continually optimised that generate the best high quality outputs. And at that point, you're not even prompting, you're just feeding a model a string of numbers because you know, this is the string of numbers it tends to generate the best search results are response to a customer query. So how does that work over the course of the next few years and to what degree is it important that the information that goes into the language model is extremely easy for a person to understand as long as the outputs are good enough? We'll have to see. That might be something that's very use case dependent, where actually in some cases, it's fine. And other cases, we really, really need to know what was asked, or what was requested such that we can be confident on the outputs.
Tracey Follows 30:16
And so how do you think AI collaboration in this space is going to work? I was gonna say, in the workplace, but they're not workplaces anymore are they? Because we're not in workplaces where we are, but they're not just the office. Do you have any thoughts on this about the new skills, I mean, because you've kind of touched on it a little bit, the new kinds of ways that the modes, or the skills that we will need to adopt in order to integrate this technology into what we're doing?
Josh Muncke 30:40
I think one of the really interesting things that you can see right away, is this idea of kind of co collaboration and co pilot co creation with these models. And so the skill that that really necessitates is, is kind of thinking out loud, isn't it? It's an ability to work in a way where you make an initial suggestion, you can articulate what you want, without precisely knowing how you want it, get the results from the model, and then iterate and provide feedback. And that idea of kind of working out loud, obviously, you know, via a text interface with a machine, but you're still kind of working in a slightly different way than you would maybe just on your own in front of a laptop, or a terminal or a phone. So that kind of co creation, that idea of a co pilot, for most people in most jobs is a pretty different way of working than they would of course, you're used to working with people as part of a team. But that's not quite the same thing, when you're producing an output individually. And so that idea of how you engage with an agent in that way is pretty new. And I think that's something that I think will shift very, very rapidly. Just as we saw children and teenagers, almost overnight, become completely familiar with how to use an iPad and an iPhone, it was just a very, very rapid shift towards something that was clearly very useful. I think that as we see these tools, and these models get baked into education, training, which they absolutely will do quite quickly, I think we will very quickly see people starting to work in a different way, by using these tools as a co creator, co collaborator, co pilot, and that applies to whether you're a software developer, a data scientist, a copywriter, a marketer, you know, these are all places where people will need to have that particular skill. More broadly than that, yeah, you know, who knows, because these models have so many uses, so many use cases, the way that we're going to use them in different places is going to look different. And so the skills that people will need, in terms of how they interact with them, or maybe don't, you know, is, is kind of up for grabs, I think.
Tracey Follows 32:41
What would be a good example of a problem today that is really like facing us now that you would be advising a client on, for example?
Josh Muncke 32:48
increasingly, if we were to use these models to play an important role in, let's say, a risk management process, or a customer service process, then there is a real risk that if the model gets an answer wrong, but that looks plausibly correct, that could present a genuine risk, potentially a safety risk to an operator, or a commercial risk, right, by giving incorrect information to a customer. And so it doesn't need to be existential to be problematic and dangerous from the perspective of a business or a consumer. And so when we think about like hallucination that gets you into the kind of questions about the types of use cases that you want to use these models for. So even just being selective about where you use them as the first question, really, when it comes to kind of thinking through safety. And then clearly how you put in place the right processes, safeguards validation, human in the loop, around the light language model, such that you are not just allowing the model itself, however, convincing implausible, the outputs look, to kind of autonomously run a process end to end but you're actually making using the powers of the model but also using the kind of validatary process that a human can play, to ensure that the outputs are correct, high quality, easy to understand, and are not presenting an undue risk. And so that a lot of what we're doing in that safety space with large language models is actually thinking through those kinds of problems, as opposed to thinking about whether or not there's a risk they might escape into a kind of a, an uncontrolled runaway AGI type environment.
Sam Gregory 34:25
I'm Sam Gregory. I'm Executive Director at the Human Rights Network called witness and we're gonna be talking about deep fakes and AI today, so I probably need to sort of back up and explain why a Human Rights network would would care about this issue. Witness was created actually, almost 30 years ago after an incident of police violence in the US, the Rodney King incident, which is when a black American man was assaulted by the police and it was captured on camera. And basically over the last 30 years, what we've done is evolved with the evolution of how both professional human rights defenders but ordinary people, capture video, tell narratives about critical human rights issues and civic journalism issues all around the world. And what that inevitably meant over time is you also have to really defend the integrity of those accounts, right? They get challenged people say that what was filmed isn't true, they claim that it's been manipulated, they claim that even the underlying truths didn't happen. And so right now Witness is an organisation that works across the world. I have colleagues on five continents. And a big part of our work is literally working very closely with human rights activists who are filming in protests, documenting war crimes in Ukraine, filming land rights abuses in Brazil. But what we learned over time was that you also had to really think about kind of the tech infrastructure that set the terms for how, you know, an ordinary person could create images that could be trusted, could share videos that people would believe. And so about five years ago, we started to see the advent of what at the time was known as deep fakes. These ways in which you know, you could swap, you know, someone's face or manipulate a video in increasingly sophisticated ways. And so we launched an initiative called Prepare, Don't Panic, which was, I think, the first one in the world to really say, how do we start to prepare for this potential threat, but do it in a really inclusive global way, that centred people who actually already have experienced similar harms, right? So if you're a human rights activist, you know, in a country like Kenya, probably already someone has tried to fake your image in a photo. Someone has already said that your story is a lie. Someone has already tried to disprove your account. So let's start with those folks to think about how we start to structure an approach that might also be super relevant for everyone else in the world who is going to face the harms of these as they get more widespread.
Tracey Follows 36:38
I think it's really interesting that people think that they can spot deep fakes to an extent these days, because in a funny sort of way, we've been educated, but to an extent. So everybody thinks of the archetypal sort of Tom Cruise deep fake and oh, that's a deep fake, isn't it? But as you point out, it's much more widespread and much greater scale. I wonder if you could take us through some of the types of I don't know what to call it really manipulation, I suppose of all kinds and where you see it cropping up most?
Sam Gregory 37:10
Yeah, so yeah, deep fakes is a confusing word. Because I think for most people, yeah, it kind of summons up the idea of the face swap, right, you know, you've swapped Tom Cruise's face onto the face of a very talented mimic, and you have those incredible ones we've seen online. But that's really only the tip of the iceberg. And, and in some senses, that's also the part that is still hardest to do, right? It's still really hard to really make a completely convincing deep fake of, say, a well known person in a way that really fools people. So you know, it's actually the Tom Cruise example is quite a good one. Because under that you have a really talented professional impersonator of Tom Cruise, Miles Fisher, you have a really great company that knows how to do visual effects around it. And, you know, it's, it's, you know, it's, it's pretty convincing. And, but that's hard for perhaps the average person to do and I think it's worthwhile stepping back and saying that, like, deep fakes are part of a field that people talk about sometimes as synthetic media. And it's part of this bigger field of AI, or intersects to this field of AI called generative AI, which is sort of the buzz phrase people have heard, like, in relation to like ChatGPT, or tools like Dali, and Midjourney. And this whole universe allows a bunch of things. So, you know, at the very simple end, it's about things that have gotten much easier in the last year or two. So you know, you can take a photo, and you can animate the photo, right? And many people have done that, right. Like, I did it with my great grandmother, I colourized, an old photo, and then I made her, you know, move and say a few words, right. And that's got very easy, because it no longer requires you to sort of train it for a specific person, it just often requires one image to do it, right. So these are some trends that have happened as well as to make it easier not require you to have lots of images of say, the person you're shifting. So the very simple end is, you know, ways you can take an image, you can make it move, the kind of commercial things we've seen in a tool like Adobe Photoshop. You can, you know, do things that you used to be able to do with photo and you can now do with video, like, you know, remove a moving object in a video in the same way you could pull out, you know, something you didn't like in a photo using Photoshop before. So those are really simple things that have been commercialised. And then you have things that are really on the cusp of getting much more mainstream. And often people talk about things like lip sync dubbing and puppetry, which are these ideas of you can either make someone look like they said something they didn't did. So you match the lips of someone to either, you know, a voice mimic or maybe a voice clone and you make them look like they said something they never actually said in reality, right. And that that's something that is being commercialised very broadly. And I can talk about the risks of that in a second because obviously, that's actually more subtle in some ways than a full face swap. It's, you know, like you just see someone saying something and you're like, oh, they said that. So that's been happening. And then there are other ways you can do this sort of puppetry, right? You can make someone move in a way they never did, right? You can make someone look like you know, in a funny way, like they're dancing, you know, and they never did, right. So there's a whole range of ways of sort of puppetry that are getting much easier. And they're often being combined. And I sort of pointed to this by talking about audio without getting easier to fake audio or to clone audio, right, which is also something that's progressed pretty rapidly, right? So, you know, you can get quite short clips of someone's voice and then create a voice that is close enough that it might fool someone. And that sounds close enough to the types of environments we operate in, right, like a phone call, or maybe a noisy background, that sound more realistic than kind of that sort of clean cut, like it's a computer voice against, you know, this very neutral background. So right in the sort of centre of sort of deep fakery and synthetic media, you've got things like lip sync dubbing and these fake audio that are, in some ways, perhaps some of the most threatening areas right now. And alongside that, you've got another big set of sort of commercial industry, but also much more available tools that are being used in business to do things like create an avatar of a realistic looking person, right? Like, maybe it looks like a news anchor saying something. It's not a real person, but it looks like a real person. And that sort of leads us into perhaps the final area of what's been happening, which is really closely related to this area of so called generative AI, which is the ability to create new pieces of media, from existing data of events or people that never existed, right. And the place people are familiar with that, over the last few years is probably, you know, encountering fake faces, right of people who never existed. There is a website, this person does not exist.com, where you could just, you know, create endless faces of realistic looking people who didn't exist. What's changed has been these generative AI tools. And the best known ones are like Dali, Midjourney, the tools built from an open source set of code called Stable Diffusion. And what those allow you to do is something known as text to image. And text to image is basically, you write a sentence, and it produces an image of that sentence, and then potentially endless variants of that. So I might create a sentence like, as we saw last month, something like I'd like a photo of the pope in a puffer jacket, make it Balenciaga, right, right? You know, and then you can generate this, and this is a big shift. And there's lots of implications of the shift with text to image and soon text to video, because it makes it much more accessible, much easier to do to create realistic looking images of often real people doing things they never did.
Tracey Follows 42:18
Yeah, it's fascinating. And there's so much bit around it. It's interesting you mentioned the Pope, actually, because that image, there's something very specific about that image. It's almost like it's that thing of something being telically possible. We know it's not real, but we kind of hope it is real, and we'll treat it as real. And I think that's some of the reason this stuff gets passed around in such a widespread manner, even if we're not sure. Or maybe we know. But it's a good laugh, isn't it? Or it's sort of it's a joke to pass around. But it's not really a joke if you're on the other end of it. And I wondered what your point of view was on who's responsible? Because I remember was it was pre pandemic, so it must have been like 2019, something like that when Macron was tweeting that photo image of the Brazilian rainforest and attributing it to the wildfires, and actually afterwards, only it was about three days after I think people were saying, well hang on a minute, this, this photo image is 16 years old. And it's nothing to do with what's going on now. Now, I'd love your point of view on whether celebrities, politicians, people like that are just as responsible or irresponsible to pass this stuff around as like the everyday person because they've got the big accounts, and they're often they're more trusted.
Sam Gregory 43:35
Yeah. And I think I want to pick up one point, you just said Tracey, which is actually about not sort of de historicizing what's happening here, right? Like, often when we talk about new tech, it's like, it's completely new. We don't think about who might have had experience with this in the past, who might think about the solutions, the ways we dealt with it. And the analogy you're drawing is actually, like in the work we've been doing a witness probably for 15 years. And I often deed it back, for example, to the Syrian conflict, where we saw this happening a lot around human rights imagery, people shared these so called shallow fakes. Which is like someone just takes an image they miss contextualise it. And they don't always do it maliciously, sometimes people are just trying to represent, you know, an issue that they care about. They don't have exactly the image and they share it, you know, in order to sort of demonstrate their affiliation to something, to try and incite attention, to try and promote a cause they care about. And I've had conversations with folks who've shared images like this in quite extreme human rights crises where they talk about, I knew that it wasn't exactly what it is, but I knew that it represents that right? And so, you know, and then also people who maliciously share because they're trying to incite hatred, or do you know, or deceive people, but it's a really complicated mix of motivations around how people share imagery and what it represents to them. And I think we have to understand that when we look at how people share shallow fakes and, you know, and emotional real images and of course, deep fakes, right. And so sometimes, as you say, I think people may know they're sharing something that's manipulated. People often talk about the liars dividend this idea that you kind of share a footage, you claim that something real is false. But actually often it's just people leaning into what they want to believe. And I think that's coming to your question around the role of influencers and celebrities, right? They have a tremendous reach. Right. And they sort of set the tone about how people share. And so I think there is a responsibility that sits with them. I think the challenge is that probably for many celebrities, they're perhaps in some ways, as ill equipped as ordinary people to know, increasingly how to discern it. Like I remember Chrissy Tegan, right? Referring to the Pope video, which was like, Oh, my God, I got fooled, right, or words that affected her Twitter account? And, you know, and like it's, it seems, in some ways unfair to expect that she would be able to discern, for example, you know, is she looking forensically at each image to try and work out if the Pope has his ring on the wrong hand? Or has six fingers on one hand? You know, like, so I think there's both, yes, we place a responsibility on people who have greater reach to be responsible about how they share. But we also need to be very careful we don't place responsibility on this problem on essentially the end, consumers, distributors, users, because we're least well placed to be able to do this.
Tracey Follows 46:08
So let's talk about some of the solutions then. Maybe tell us some of the use cases you've heard or maybe it's working with activist groups or journalists? Take us through the landscape with some of the solutions.
Sam Gregory 46:19
So the solutions, I think we really have to think across the spectrum, right? It's there's not one silver bullet here. It's a classic phrase, everyone says, but it's absolutely true here. One thing to think about is it needs a responsibility that goes across kind of a pipeline of how we make media, right, so you can't just blame the end consumer. Right? So I think a lot of what's happened around, you know, the fake Trump photos, or the fake Pope photos is people saying, Well, surely you could have seen that the Pope always wears his ring on his left hand or right hand, which way it is like the people signet ring. Or surely you know that hands don't do well. Or surely you know that you can't write text well in these types of tools. And the problem with that is it both assumes that people should have this kind of forensic mindset of looking at any image they see, which is unfair. I don't look at every image in my timeline or on TikTok with that view. And the second is that those clues go away over time, right? We know that those clues are just the sort of the failures of the current algorithm. So even like, for example, this tip that hands don't look great in image, you know, images created with these text to image isn't really true with some tools. And it's getting easier to write text and images and deep fakes didn't used to blink. But now they blink. Right? So when we tell people these things, we're giving them false clues that won't last, right. And that's actually very harmful, because people remember stuff people love really easy, like heuristics, right? Like, oh, deep fakes don't blink, when in fact, you know, the moment that was shared as a tip, people started making deep fakes that blinked. You know, the hands, people want to improve hands, you know, as creators of these tools, because it's an obvious thing to try and improve. It's a technical challenge to do that. And but those ideas stick in people's heads. So we can't start with blaming the users. We can say you need to have we need to double down on media literacy, and say, Look, you know, the same tools of like stopping, thinking if there are other places that would corroborate a story you're reading, seeing if there's an original image that this has been manipulated from, right, those are classic media literacy strategies that we should still have. But the point is, those need to be supported by the pipeline that comes before that, right. So you know, if you that what I mean by that pipeline is there are people who build the underlying models for this literally the kind of ways in which the technology, the sort of foundations, the technology is structured on. There are people who build tools on top of that, and there are people who create distribution, right. So if, for example, you want your AI to be able to understand that a piece of media was synthesised, you might want to have a watermark that's placed at the model stage that is visible all the way through. You might want to have what's known as authenticity, and provenance infrastructure. That's ways in which you can show that a piece of media was edited in a particular way, was distributed in a particular way, that you could look at and know Oh, I know that this was, you know, a photo that was shot, you know, on the streets of Chicago last year, and then someone did a face swap in it, and then they shared it on Tik Tok. Right, you know, so those are the types of signals that could help people make decisions on this through a pipeline that goes, you know, across this, and there's some core principles you'd want in those types of technical solutions that are around, yes, disclosure, but also consent and privacy that you need to build into how you do that. Right. So those are the kinds of sort of signals we might want, across, you know, our kind of media pipeline so that it's not just us guessing when we see a piece of media. And I think it's interesting, I think we can also think about that as being part of sort of the next wave of creativity and creating media. It's like, I often look at the types of videos I have in my, you know, TikTok for you page or in my following. And if you look at that, you already see that media is much more visibly constructed than say it was 10 years ago, right? You see, like someone's got an AI filter. They've stitched it with something, they've, you know, they've edited in particular ways. So actually, I think we're increasingly comfortable with knowing that our media is very constructed. And so actually we can be much better at saying, Actually, let's just be much more transparent about how media is made. And that might be this was made with AI, this was made with a mix of AI and real footage, which is going to happen all the time. This was made based on this data set, right? Like, I think we can live with a bit more complexity. But to do that, you have to have the pipeline. So that's one set of solutions is kind of like the technical ways you can do this. You know, the other is like, what are the things we do not allow? And we do not permit to happen in these types of systems because we believe they're illegal or within democratic systems otherwise, right? So, you know, should it be legal to create non consensual sexual images of women without their consent and distribute them? Right, like, that's a place where it's like, we should have legal safeguards, that, you know, make it very hard for people to build tools that are oriented towards that, that criminalise people who do that in a commercialised way, or do it in a way that is used maliciously. And again, there's a whole debate around how to think about intent or not in these ones, right. But like we there are places where we can say, Look, we need to really get strong legislation that that addresses something that we agree is a harm. You know, similarly, there are some laws, that are starting to happen around election usage, right, under what context. Should you be able to use this in, you know, in elections and those types of contexts? There's a couple of places where this is happening. You know, I think that's all against the backdrop of like, the bigger picture of AI legislation, right, like, what are the big safeguards on transparency on this? Like, do we know what's happening under the hood of these models? Do we know that there are going to be responses if there are widespread harms? Because in some sense, what I'm talking about here are individual media items, right? How do you recognise the Pope in the puffer jacket, but there's a bigger issue here, which is applies to both images and video and text, right, which is kind of this idea that maybe our whole information ecosystem is going to be polluted with fake images, fake videos, some of which are hallucinations. This idea that like sometimes these systems create false facts, or false information that looks like facts, right. And so that's a bigger issue of like, the bigger sort of ecosystem that can't be addressed at every individual content item. That's a part of it. But it's a bigger picture of like, well, actually, how do we want to regulate these whole systems? And what are the safeguards when a whole system starts creating information that's deceptive, false, you know, and undiscernible? Right. So I think we have to think at multiple levels, like the consumer, the pipeline of the technologies, the individual content item, but also this bigger picture of kind of the ecosystem around us.
Tracey Follows 52:25
See, as I hear you talk, I'm thinking how much, what percentage of our media experiences will be real in the future? I mean, will any? Because it could be that the vast majority are either exactly what you've just been explaining these imagined futures and speculative fictions, in a sense. And also they could be the suppression of information, which again, creates a misrepresentation of reality. I mean, I think that's one of the things I'm most worried about, actually, the suppression and the missing information, not misinformation. We've seen it through the elections in recent years, we've seen you know, people disappearing out of photos, anything that can be digital can be disappeared. And I really do think it's a big problem, because it's not like you can access it, it's not there. So you can't access it to even have a debate about it. I wondered what your thoughts are on that?
Sam Gregory 53:18
Yeah, I think there's a number of trends, including the one you're describing, that are important to think about when we're thinking about kind of how much is real, how much is fake. And I also want to I want to push back a little bit on the real and fake because I think we're going to be in a world there's much more sort of mix. And we have to just be better at interpreting that.
Tracey Follows 53:35
Well, it's all one thing eventually, it's all a real fake, genuine fake world. And we were creating those ourselves with profiles there. They're us, but not us in a way. And we were trying to navigate this liminal space. So I know what you mean, I do agree. It's just the only way I can describe it is at the moment is this distinction?
Sam Gregory 53:53
I think it's I what I'm sort of thinking about there as like, you know, when we create a photo, like we already use AI to like, you know, improve our faces, make ourselves smile, but put the bokeh effect in the background. So like, sort of naming that, because I think often we get these statistics, like 90% of things are going to be synthetic by 2026 is a common one that's named. And I'm like, I'm not sure how helpful that is. Because it sort of depends, is it the 90% we care about? Or is it the 90% that we want to be synthetic, right? Like, if it's 90% of my TikTok feed is synthetic, I'm kind of already expecting that. And I kind of want that, and I'm enjoying it. So I think like just knowing that numbers, and the sort of the the real/false is going to be complicated and also will be used against us, right? Because it'll be like, Well, you can't believe 90% of stuff when in fact, we might want 90% of stuff to be not exactly real, in some sense. Yeah. So but I think that there's some trends that are important and you're naming on. It's like, will it be easier to kind of erase things? And I think it's true that like this allows us to erase pieces of content, right? It's easier to manipulate discrete content items. I think there are some other things that are sort of around it that go to the same worry you have right? One is the idea that this also makes it easier to personalise realities for particular people. That very much this is about like personalised information, you can already see it with like the ad some of the ideas for using generative AI and ads are about personalised ads, right? You know, and that has a real pernicious effect, because you can personalise in ways that reinforce your biases, that put you in even more of a bubble of one, right. And so, you know, if we're trying to think about how we share information, how we engage each other on a shared platform of actual information, the more we're personalised, there's a risk that we're sort of, we're reducing that. The second is I think that one of the sort of ways in which this potentially changes our information environment, it's particularly to do with some of these trends that are happening with the accessibility of these tools is the ability to create volume Right. And so, you know, we could think about that in, you know, in the ways we might think about very organised strategies around trying to deceive people, right? There's this idea of floods of falsehood that comes up in, you know, disinformation discussions, which is like, you can overwhelm an information environment with lots of contradictory accounts, and people just throw their hands up in the air because they have no idea what's true. So volume is easier in this environment. I also just think there's a volume creation thing that makes it harder possibly to find the things that you might want to be finding, you should be seeing, right like, and we definitely hear that from human rights activists, they're like, I can't create 60 different accounts of you know, that that incident that happened. There is only one account, right, and it's the important one, but an a, you know, an act, an opposing act to be they a corporate actor, or a government actor, could create 100 Different contradictory accounts. They have no ethics about this, they can create 100 stories, and I have just my one story that really matters. Right. And so and they might also try to erase that story, right, they might try and release a version of that story that has, you know, removed, you know, the police officer who is assaulting a protester, right. So all of these kind of come together. And meanwhile, lots of other people might be in these environments, which are very personalised and bubbled where they really are just living in a mixed reality world, and other people are throwing up their hands because they like so many false and true accounts, I don't know what to believe. And, you know, I'm listening to, you know, a Chat GPT or equivalent, and I know, it hallucinates. And so you can't even tell when it tells you an answer, whether it's true or false. Right. So I think there's a lot of factors here. And this is really about the bigger information ecosystem, where we need to go well, how do we think about those implications? You know, both to protect individual, and maybe, you know, group actors who really want to deceive us, or have an interest in maliciously deceiving us, or avoiding accountability for their actions. But also just more broadly, like how we may all be in a place where we are more confused, less confident in what we see. And then these critical voices who we really perhaps should trust are the ones who have the hardest time breaking through the chatter.
Tracey Follows 57:33
It's good to spend as much time as possible, I think, in the future with real human beings just to like, keep re-anchoring ourselves into like, the physical. I'm not gonna say real, the physical world. I think it's going to be more and more important. Just as we wrap up, and you've covered so much. And it's also fascinating. I wonder if you want to just tell us a little bit about what's on your horizon? I could I ask you, where will we be by 2030. But I think he's at least impossible, really to say right now. And things are moving so fast. And as you say, the speed and the scale of everything. But what are some of the trends you think are reaching out into the next few years that we should I mean, I'm really worried about voice. I mean, I'm really worried. But I've seen what 11 Labs and the like are doing, I think, I spoke to the bank the other day, I rang up the bank. And I go through, obviously, voice biometrics, and like we say your name, say your address. And I said to her this, I really do not feel confident about this technology anymore. And I started to talk to her about this. She said, Oh, it's okay. Our systems, you know, you can't override our systems. The naivete of it is just incredible. So I'm quite worried about voice. But I'd love to know what you're worried about and looking at on the horizon.
Sam Gregory 58:47
Yeah, so the first thing to say is, it's quite hard to predict, like long trajectories, even medium to sort of even medium term, right. I think that sort of generative AI explosion of the last six or eight months probably came faster than myself and others expected. And, you know, I'm in the US context. And people are always asking, What are we going to do in the 2024 elections? I'm like, that's 14 months away, or 16 months away. I don't know exactly where we'll be. I think there are things we can point to and I agree, I think there are technology trends, where you can see trend lines. So audio has gotten a lot easier to do. And we're seeing it used in very common everyday contexts, like you're describing someone fooled actually the Australian National biometric system recently. We're seeing scam calls being used. We're seeing swatting calls in the US where it's an automated voice right calling to do swatting on folks. So audio is improving rapidly and I find very worrying also looking globally we've seen a lot of misuse of audio. It also lacks all the sort of semantic clues around it right a video like this so many things you would look at to see if it's wrong while audio you're just kind of listening and you're going really hard is it it's a little too electronic right? It's there's just no surrounding clues particularly for example so like when it's for example shared and a WhatsApp group, as in many parts of the world, where voice messages in WhatsApp are like you know how people communicate. So audio is improving rapidly. These text to image and text video tools have been improving extremely rapidly, right? Like I was even describing, like the progress in the image generation that's gotten like in the way to generate hands. Text to video still looks kind of funny if you've seen that, right? It's like, you know, like, you know, Will Smith eating spaghetti. But it's moving fast.I don't think it's like at the speed where we're going to have like full length, you know, complex, deceptive videos, all that super soon, right cautious about my words, but, but those have advanced really rapidly. You know, the ability to create realistic looking avatars that say things advanced really rapidly. So all these tech trends are advancing. It still remains somewhat hard to do a super convincing face walk deep fake in a kind of real time context. So if something happened this afternoon in New York City, that was like where I wanted to face swap someone into it, and have it ready by the next morning, I would still be a little cautious about when that's possible. So that's the place where it's still like, you know, where a lot of people's fears sit. But actually, probably the realities is the day to day audio, text to image video that never existed. And the non consensual sexual deep fakes that continue to proliferate, get easier and easier to use. So that's sort of the tech trend. The trend lines on things like regulation - suddenly everyone's paying attention to this. You've got proposals in the UK, you've got proposals in the US, you've got the EU pretty close to final stages on the EU AI act. China just passed or sent for comment, but it'll pass a set of regulations around this, you know, and I think there's, you know, we'll see regulation in the next couple of years. And the critical question is, will it address adequately this pipeline responsibility? You know, and will it create collateral harms, like, I spend a lot of my time working with my colleagues who are around the rest of the world, and often you see legislation that's kind of copycat legislation, you know, to suppress journalistic freedoms to suppress satire to suppress free speech that is used in response to these threats. And we even seen it, we saw it around COVID. We saw it around, you know, the the fear of deep fakes, right. So I think regulation is important, but it really needs to go to the core of the problem, right? Like the transparency, the regulation of these models, the real attention to very specific harms, the accountability of tech developers, and it needs to really think as it sort of proposes infrastructure solutions like this authenticity solutions that it doesn't do that while compromising privacy, for example. Right. So I think the tech trend is in sort of the regulatory trend is action, action action. And the question is, how do we make sure that's actually addressing the problems, it isn't creating the harms? You know, the bigger picture like I worry very much around this kind of just both the individual content undermining critical voices in our societies and attacking, you know, ordinary people, and also critical truth tellers, but also, this broader undermining of kind of our epistemic trust, right, like this belief that, you know, we have, you know, and I think, you know, you can approach that in multiple ways. Let's say, from our own, you know, witness perspective, we're saying, you have to fortify the truth. And some of that might be tactical, literary, like film 360. In a critical event, some of that might be infrastructure, like having a tool that can show how a piece of media was made. And some of that has to be, you know, policy side, right, like protecting people's right to film in public spaces is important, right, so you actually have the literal documentation. And it's also going to be like the types of AI regulation that actually structure a space, that still, that doesn't suppress speech that allows people to be trusted, that doesn't undermine this shared ecosystem that we still need to aspire to. I don't think we can give up on the idea that we should be able to communicate with each other in a shared space.
Tracey Follows 1:03:35
I was just thinking about when you were talking about media literacy. And I would say it's even more than that. In addition, it's media forensics, like in terms of like, almost putting it on the curriculum. Like it's almost one of those 21st century skills that people are going to have, but the question I have in my mind is, are there enough people to teach it? Where do we start?
Sam Gregory 1:03:56
This is, I realised I didn't talk about this, because one of the things we've spent a lot of time working on in, in Witness has been around detection. So like, I've been talking a lot around kind of like media literacy and authenticity. But like, obviously, people also ask like, how do we detect this? Can we run a tool that shows you something's manipulated? And there's sort of multiple problems around that. One is that the tools aren't all that accurate, they don't work very well on kind of social media, video or photos. And they're not widely available, and the expertise around them is not widely available. So, you know, we often talk about an equity gap around media forensics, which is that the people who need it most don't have access to it. There's just you know, it's very much concentrated in academia, law enforcement, you know, intelligence agencies, not in civil society, not in most journalism. And so, I think we need to think about and this is a big structural challenge. How do we have more people have expertise in media forensics, including in spaces like civil society, like journalism? You know, not just in places that may have mixed motivations when they want to disclose whether something's fake or not, and you know, and are controlled by the state right versus, you know, civil society and the Media. So we want to do that. I get very conflicted about media forensics. It's interesting, because I think part of what we're doing, if we try and encourage the sort of people talk about the forensic turn in some of the work that people do, which is like looking really closely at images and video and trying to scrutinise them and you see it around Ukraine, people like going like, I'm just going to look at every pixel and try and work out whether that's a reflection in that mirror. And was that real? Was that fake? And the danger in that is like, it probably isn't that productive for most people, because they don't have the skills or the tools. But it encourages a scepticism, that is that can't get us to a place. So it's a really interesting balance. We want to have more availability of media forensics, we want tools that are interpretable that are available to more people. But we probably don't want to switch media literacy for media forensics for individual people, because actually, probably, in most cases, it can be better to say actually, Is this likely to be credible? Do other sources confirm this? You know, was this manipulated from an original versus trying to like look really closely at the pixels? So it's, it's a complicated landscape? Like as we think about media forensics, there's definitely the gap you point to, the sort of bottleneck, and we're going to need more of it. At the same time, we probably want to sort of get a right balancing act about how much we encourage individual people to be media forensicists so the sound right does it forensic experts versus you know. So it's an interesting balance
Tracey Follows 1:06:24
Thank you for listening to the Future of You, hosted by me Tracy follows check out the show notes for more info about the topics covered in this episode. Do like and subscribe wherever you listen to podcasts. And if you know someone you think will enjoy this episode, please do share it with them. Visit the futureofyou.co.uk For more on the future of identity in a digital world, and futuremade.consulting for the future of everything else. The Future of You podcast is produced by Big Tent Media.